Risks and Rewards of Big Data
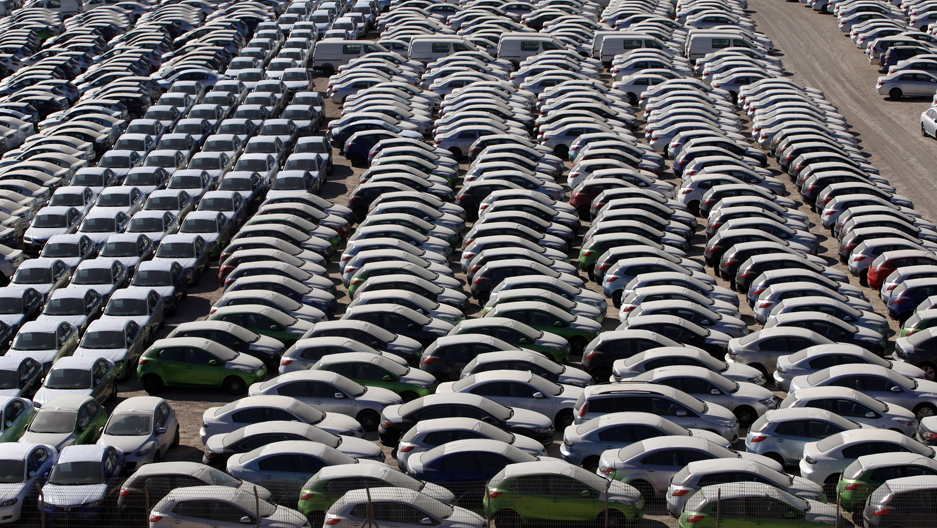
Big data holds enormous promise. With big data platforms and analysis tools, companies can getter understand how their products are being used, tailor them to their clients’ needs, make them more efficient, and predict trends. Despite these benefits, there is cause for concern as some of this data—on an individual and aggregate level—can contain personally-identifiable information and can be collected by hackers, criminals and foreign governments.
Microsoft’s Elizabeth Bruce, IBM’s Dan Chenok, and Andrew Hilts, the executive director of Open Effect, join CFR’s Karen Kornbluh to discuss the risks and rewards of big data. The panelists examine risk to privacy in a big data world, whether our notions of privacy should change, and whether the benefits of big data collection outweigh the privacy consequences.
SPEAKERS
Elizabeth Bruce, University Lead, Technology and Civic Engagement, Microsoft Corporation; Former Executive Director, Big Data Initiative, Massachusetts Institute of Technology
Dan Chenok, Senior Fellow, IBM Center for the Business of Government
Andrew Hilts, Executive Director, Open Effect
KORNBLUH: Hello. Welcome. Welcome to the panel “The Risks and Rewards of Big Data.” We have the perfect panel to address this issue: Elizabeth Bruce is at Microsoft now, but she was the founder and executive director of the Big Data Initiative at MIT; Dan Chenok is the executive director of the IBM Center for the Business of Government; and Andrew Hilts is the executive director of Open Effect and a researcher at the Citizen Lab at the University of Toronto.
We’re going to be talking about the risks of privacy in a big data world, whether our notions of privacy should change given the explosion of metadata our digital devices produce and whether the benefits of big data collection outweighs the privacy consequences.
We’re going to have a discussion for about 35 minutes and then open it up to your questions for the remaining 25 minutes. If you see me looking at my watch, it’s just to make sure we stay on that schedule.
I thought we would start with an overview and just figure out what big data is and what some of the risks are, and then maybe we’ll turn to some solutions.
And so for that, Elizabeth, what is big data? You know, what are some misconceptions about it? And why are we all using it? What’s it good for, what could it be good for?
BRUCE: OK, thank you. And thank you for having me. It’s great to be here. So I’m going to speak from—we started the Big Data Initiative at MIT very much focused on helping enable and build the technologies that could enable big data. So big data is really, from my perspective, a—the real value comes from massively increasing our power of observation. And this can be around physical things. You think about the internet of things, you can now massively track pretty much anything you want at any time.
It also increases our power of observation of human behavior in a way that was never before possible. So if you think about social media, social network sites, right, you have the ability to understand, even your phone as you carry it around, to understand human behavior at a scale. And if you bring those two things together, if you look at some of our biggest challenges that we face, whether it’s in health care, whether it’s in finance, whether it’s in cities and dealing with congestion and all the challenges of access to resources, I think there’s a lot of excitement, certainly in the research community, with people that think far ahead that big data will provide us an amazing new tool for seeing and understanding these very complex systems.
So if we take—so that’s very exciting. And there’s a lot of, like, profound things there, right? Big data and you call it data-driven research is something that we’re very much at the tip of the iceberg. So when people talk about big data now, what you often hear about are the things that you see, like recommendation engines, right? Whether it’s Amazon serving you products that you should buy, it’s a lot more targeted advertising, it’s, you know, Uber matching products and services. There’s a lot of—there’s a class of machine learning that’s recommendation engines. And that’s about doing a much better job of fitting products and services to your individual needs or desires or anticipated desires, so that will continue to increase.
Let’s see. If we take something—step back from, like, the consumer world for a second and say, well, this isn’t just about serving ads. Let’s think about some really profound, like, ways it’s going to improve lives. There is an example, and I’m sure you have examples, too, from other areas, but health care. So there’s researchers at—and I’ll just give one example where I feel like it’s the power of big data to massively increase our ability to observe things.
If you are in a hospital today and your doctor orders and EKG, and you’re running the EKG and you get streams of data that just come off and the doctor look at it, and it’s a signal, and he says, yep, that looks good, or no, that doesn’t look good, and sends you off for more tests. So what some researchers at MIT did is, like, wow, that’s a lot of data that falls literally on the floor. We don’t record all of that or analyze it, and we certainly don’t record and analyze that over huge amounts of patients. So they started a project where they took all the EKG data, recorded all the data, looked at different patient populations, and in the end they were able to discover anomalies in that data at a very particular, fine-grained level that correlated to higher probability of certain heart disease, you know, heart failure. And that’s profound, like, that’s a whole new way of predicting or assessing someone’s risk of having a health problem. So that’s, like, one specific example, that you can take that across a lot of medical data. And of course, that’s the power of electronic medical records.
Now that we’ve digitized so much of this information, digital images, our genome, our, you know, our patient medical records, all of that, lab tests, all of that information can now be brought together in ways that it’s never been brought together before. And the idea is that things like a particular disease you can now analyze that data across different patient cohorts and look for new biomarkers and new ways of perhaps detecting and treating diseases. So that’s really profound.
There’s another example that I like in transportation. So it’s not just D.C. I had trouble getting to dinner on time last night, but everybody, every city I go to thinks they have the worst traffic. And that’s just in the U.S. You know, a lot of developing countries, as you look, the world’s population is increasingly going to be in cities, and we’re dealing with congestion issues everywhere. So everyone talks about transportation issues.
The solution isn’t just technical in a sense that you’re going to come up with autonomous vehicles, and everyone is going to, you know, just switch to a different vehicle. It’s not going to be just mass transit, right? It’s going to be a complex solution that also has to do with human behavior.
So let me back up. So in a city like Nairobi in Kenya, there was a researcher at MIT who looked at the city. And they have their informal bus systems, matatus, that drive around the whole city and bring people, you know, move people around. It was incredibly ad hoc, incredibly informal, and there was no map. And so what this project did was they said, OK, everyone donate your data from your cell phone. And so for a period of time, a year, they collected all this cell phone data. And then at the end of it, they could analyze it and create a map of the entire bus system. So when you talk about the power of observation, something that you could not have created before, was created from cell phone data.
Then the city of Nairobi adopted that as the official transportation map for the bus system. It’s a completely different way to create a bus transportation system map. And it’s now officially on Google. So those kinds of things are really interesting.
If you take now your Google map and you think about how we use it, we’re really excited that our Google map can show us where the traffic is and reroute us, right? But if everybody gets rerouted that way, you just create more traffic on a different route. So the future is going to be, OK, if we use everybody’s data, you now want to create dynamic routing, right? And what does that mean? You also want to look at the economics of this. And how do you incent people to drive at different times? And that incentive system can also—it can be a fine, or it can be a, well, today you don’t need to get to your office until 11, so you can go at 9. And then somebody else needs to get in, they’re willing to pay and go on a faster route. Like, think about the sort of interesting dynamics that we can use through big data to resolve issues like congestion.
KORNBLUH: That’s terrific. And so now we’re going to give you a little whiplash, and Andrew’s going to tell us about the risks of big data.
HILTS: Sure, I’d be happy to. And it’s great to be here with all of you today. So I think, you know, one of the big risks that has been alluded to earlier today has been around the use of algorithms for, you know, purposes that could potentially reinforce existing biases, whether that’s in, you know, the data that’s being collected to power those algorithms or in the design of the algorithms themselves through the biases of, you know, the computer scientists or programmers who develop them. And we see cases of such biases, you know, from time to time in the media.
And so, for example, a case that came out just recently is the ProPublica did an investigation in the use of predictive scores of convicts to their likelihood to reoffend. And in their investigation, they found that white convicts were far more likely to be assigned a low score of likelihood to reoffend, whereas black convicts had, you know, a more evenly distributed across, you know, the risk intervals. But in looking at the actual data of who did reoffend, they found that, you know, there was quite a few white convicts who were rated as a low likelihood to reoffend who did in fact reoffend versus a far fewer percentage of black convicts who were rated low as risks, they did not reoffend. So this was an example of an algorithm that was being used, that seemingly has perpetuated racial biases. So that, I think, is a significant risk.
And we, I believe, as, you know, regulators, researchers, developers of tools, need to develop a culture and internal processes where we can account for these biases as best we can and hopefully be transparent about, you know the process of designing the algorithms and ideally, you know, publicize the algorithm, how it works, so that it can be scrutinized, especially if this algorithm is being used in, you know, judicial purposes or things that can actually have tangible impact on people’s lives. This is, you know, quite serious, and I think the algorithms that are being led—or being lended sort of a veneer of objectivity need to be scrutinized in the same way as, like, any other, you know, use of evidence in criminal proceedings or things like that.
And I think that sort of veneer of objectivity is a larger cultural concern, you know, in present day. We see, for example, recently Facebook has been reported to potentially be violating the law by using a so-called ethnic affinity selector for its advertising so that people could target ads based on ethnic affinity, whether that’s, like, African American or, you know, Southeast Asian, et cetera. And this had been used for, you know, housing advertisements and potentially be used for job advertisements. And so this would be a violation of, you know, civil rights and the Fair Housing Act and so on.
But I think Facebook in its public positioning has been positioning itself not as a, you know, a company that is a media company or that can, you know, advertise things necessarily. It’s primarily a technology company, and technology is fair, and it’s based on science, and there are no, you know, inherent biases in this. I mean, not to say that, you know, people working at Facebook believe this, but that’s just been the public messaging and the discourse.
So another example with Facebook, not to pick on them, is that with the latest election there have been, you know, discussions about the fake news that has been surfacing on the website. And you know, that’s being powered by Facebook’s recommendation algorithm. It’s something that people are engaging with and liking, but it’s been showing up in, you know, Facebook’s trending topics section. And, you know, earlier in the year, Facebook came under controversy because it’s trending topics section was, you know, supposedly biased against conservative news sources. So in response to that, Facebook, you know, removed the human editors who were, you know, curating these trending topics and instead be like—they said, basically, don’t worry about it, the humans are out of the equation, we have an algorithm now taking care of it. So, you know, no bias anymore was the, you know, inference.
And I think that notion that technology is inherently neutral needs to be questioned more thoroughly in society. And I think that’s probably sort of the underlying risk that emerges in sort of this big data age. And I can—you know, again, to other risks as well, but I think that’s sort of a foundational one.
Associated with that is the notion that basically it’s sort of the premise of big data is, you know, to have a critical look at it, is that more data equals more truth equals, you know, a better society. And there’s sort of this collect-it-all mentality and we’ll figure out how to use it later. So I think, you know, it’s very much true, as Elizabeth was saying, that, you know, you can come to all these new findings by having this dataset that you wouldn’t even think about before just by analyzing it and sort of inducing patterns and things like that. So that’s not to say that it’s not valuable, but how we collect the data, how we retain it, for how long we retain it, for how long we retain it, I think that’s very important and needs to be, you know, carefully considered.
I think the reasons for the data collection need to be considered very carefully before, you know, sort of a proverbial vacuum cleaner is put in place and all this data is gathered. I think, you know, we need to have strong processes to decide what’s worth collecting and how are we going to store that and manage it in a responsible way.
KORNBLUH: Well, as we get into some solutions, Dan, maybe you can talk about what’s been done in the past and how governments and businesses are thinking about it in the future. And I just want to note in that vein that Facebook has made announcements about both racial targeting for these sensitive ads in housing and employment and so on and also on the fake news. So, you know, we can talk about some of these things as we go into solutions.
CHENOK: Sure. Thanks, Karen. And thanks to my fellow panelists for doing such a terrific explication of the issue. I look forward to the discussion.
So in addition to my hat as the leader of an IBM think tank that works with governments around the world, the Center for the Business of Government, I’ll wear a little bit of my hat as the chair of the DHS Cybersecurity Advisory Committee under the Data Privacy and Integrity Advisory Committee, and my former hat with the Office of Management and Budget here in the U.S., which is the leader of the OMB IT Policy Office in the ‘90s and 2000.
So I think that, as you say, a lot’s been done before. There is a lot of new technology here, but there’s a lot of, as I’m sure many folks in this room are aware, good practice that’s been exercised by government and has been learned over the last couple of decades around how to address evolving data standards and technologies that enable sort of massive capture, analysis-sharing, distribution, action on that data. We call that big data, but I think the same could be said for the move toward open data, which sort of creates big data in a different form that may be more accessible to citizens and providing even greater opportunities for transparency and access where you don’t have to collect things in a large, analyzable database, but you can basically grab data from lots of different places and come up with a similar solution without getting into some of the privacy issues that you’d get if you collected it all in one place, whether that’s in government or in industry or a data broker, et cetera.
So some of those elements that we’ve been talking about for a number of years include privacy by design. As you’re setting up the system, you can build in controls to both monitor the use of the data that comes in and out of that system as well as the user’s ability to access that data. That looks very different in sort of a big data machine-to-machine exchange environment than it does in an individual bilateral relationship with a company or government agency about their data.
So understanding how to basically build in policies, whether it’s a company building in policies for customers or a government agency building in policies for citizens that they’re serving, finding ways to enable that citizen to sort of follow the data through the process and understand where, when something gets shared, whether it’s machine-to-machine sharing or a large exchange across a big set between agencies or between governments, that there’s some ability to notify the individual, to sort of change how notice is done so that the individual doesn’t just sort of read a canned notice about privacy the first time the government uses it, but that the notice responsibility sort of is an interactive discussion between the individual and the company and the government as the data travels.
So I think that this concept of building privacy elements into the design of systems and the design of data flows is something that companies are working on, that the governments are learning from the private sector how to do better.
I think that this concept of the need to look at the timeless privacy principles that were discussed 40 years ago now by the OECD, by the U.S. government, by governments around the world really could use an update in terms of understanding how to apply those principles of notice, choice, transparency, access to information, redress if your information gets misused, so the ability to basically rely on big data and to open it to create transparency so that you have an understanding of where that data is and to think about it, again, more as a sort of a concept in open data enablement for the companies or governments that are making decisions from vast data stores, doesn’t have to be in the same data spot, and then from the individuals who may be affected by data in ways that 30, 40 years ago we didn’t dream about.
When the Privacy Act of 1974 was written, privacy law was basically premised on the fact that the government would do something with an individual’s data, and that something would be premised on their name. Now, as many of us know, you can put together lots of pieces of information, and those pieces can shift on a daily, hourly, or even minute-by-minute basis to create personally identifiable information that may not be the classic definition of PII, but may impact people because companies and governments are making decisions about that. And so understanding how to sort of, again, create notice about what’s happening and how the governments use that information, how companies use that information, and then enabling citizens to interact with governments and companies to be more responsible in drawing on the vast benefits, which I should have started with that.
I think that the benefits are clearly significant in sort of how data flows have evolved over the last 30 years. They create powerful examples that both of you have spoken to so well, and other examples of how citizens can really do things in society that they used to rely on governments to do. We now have the ability of citizens to report on local incidents in ways that can provide immediate response from local governments for things like traffic lights being out on their street, and that’s because we’ve got citizen reports and sensors that it can enable governments to take advantage of big data to basically array their response capabilities to go to that street where the light’s out because that street’s got more traffic at rush hour, and so fixing that light may be more important than fixing the light on the side street, even though in the old system they might have looked the same to a city traffic manager.
So the last point I’d say is that the concept of moving toward this changed notion of big data and enabling open interface also lends itself to some of the evolving systems of artificial intelligence, of cognitive computing. And I want to come back to the point about, if really used well, it can enable human decision-making. As you said, it’s not something to replace humans, but it can look across vast stores of data and help people make better decisions, whether that’s a doctor, to use another medical example, who when you come with a particular symptom in a pre-open data environment, the doctor may have relied on their own knowledge and maybe the knowledge of their immediate team, and now they can, through a cognitive system, rely on all of the medical journals that have been written, all the medical journal articles that have been written, that relate to the symptoms that they’re coming up with and ask a question to help them really take advantage of the best that’s been thought and known in the medical profession for decades to help them resolve an issue quickly and effectively. So it really helps humans make better decisions in ways that we couldn’t have imagined five to 10 years ago.
KORNBLUH: So I want to continue the conversation about solutions. But one thing you touched on, Dan, I wasn’t quite sure, I want to see if there’s a finer point that can be made. Were you suggesting that the FIPs, the privacy principles that have been relied on for so long, that those are not adequate? Or were you saying that more needs to be talked about how to apply them, or that we need to add to them?
I know the White House did this whole big data look and they said, you know what, the FIPs are appropriate, but then since then they’ve looked at this whole issue of fairness and possible discrimination. How do you, in addition to privacy by design, how do you do sort of some people have said fairness by design?
So I don’t know if you want to speak to that or, Elizbeth, if you want to take that.
CHENOK: Just to start, so I think the FIPs are timeless in their—at the highest level. I think it’s the application in how companies and governments and citizens work together to understand how to apply notice and choice and access. And it’s a different technological paradigm as to how that gets implemented when data is moving at lightning speed across the world and coming together in ways that can change minute by minute.
BRUCE: Yeah, so I agree. And the principles are all key. It’s the new complexities that big data introduces that makes it difficult to know how and what is the right way to interpret everything in today’s age. And so, if you take something like consent and transparency, that would look very different 10 years ago than it’ll look 10 years from now, right?
So everybody knows when you download an app and you click on the yes, who reads all of the terms and conditions? Nobody does. And so when you download “Angry Birds” on your app and realize someone tells you later that, oh, actually “Angry Birds” is sending all of your GPS data to “Angry Birds,” and you have no idea what they’re doing with it or who they’re selling it to, like, most users, a hundred, well, 98 percent are going to have no idea. So is that useful consent?
I mean, that’s just—and then transparency. You know, the way data is collected and then the way data is shared in a big data world is going to be highly complex. And so, how do you have transparency, algorithmic transparency? What does that mean? Are you going to ask the, you know, the coder to provide the code? I think that the ways you might interpret some of the privacy principles will be different. And I think there is a look at what are the outcomes, so you might have an—I think this is something with big data; the complexity means that it’s going to be a lot harder to detect discrimination. And algorithms by design discriminate, that’s what they do, right? They use the data, you use the data to build a model, that model is then used for new data to make decisions, better decisions. You run your new data through the model.
And so, first of all, machine learning is set up so that it continues to learn. You don’t crank out an algorithm and then it’s fixed for the next 10 years. Machine learning is about the code continuing to evolve as new data arises. And every company in the world is going to be collecting more data from more sources continuously. So you’re going to have to have a system that continues to monitor and monitor transparency of those algorithms continuously.
And then you want to look at—really, I think, some of the argument is shifting to it’s not just the upfront consent, but it’s the use. So if you look at the outcomes and you say, OK, are we having fair lending practices, then you want to look at the outcome and be able to say what’s happening there. And then do we go back to the algorithm and say, well, something’s biased? Because it may not have been the intention of the computer program who wrote that algorithm to actually bias the outcome. So it’s complex.
CHENOK: I think that point about use is really important. And it’s one that was raised. Some of you may have seen a couple of years ago the White House had a report on big data and privacy that they put out. And they talked about changing the paradigm of when the privacy right is basically exercised from collection to use, because it’s at the point of use where the individual has great benefit, but where risks can be introduced.
And interestingly as I was getting ready for the panel, I came across an Australian update to the privacy principles or update to the application of privacy principles that made a very similar finding in terms of when companies should articulate and implement at the point of use rather than at the point of sort of collection.
BRUCE: Yes. And I think we’ll be collecting a lot of data. And you sort of have seen this hype around big data where you kind of feel like it’s a Wild West of let’s just collect all the data because we don’t know what insights we might gain or what future use of that data we might have. And so I think it’s going to be increasingly difficult to monitor at the collection and the consent part.
And if you think about medical, you know, five years from now, what’s going to happen with genomic data? And, you know, if you have a disease, of course you want your genomic data perhaps to be used to help find a cure. So how do you give future consent?
And so thinking about those things, there’s also the use and then there’s the lifetime of the data. We are now in a world where we’re collecting data at a rapid rate, but we’re also able to store that data in a way that we could never do. So our children will have the opportunity to have their genome stored for, you know, a hundred years. How do you think about the whole lifecycle and when does data disappear, or does it?
CHENOK: Not to mention all their video.
BRUCE: Yeah, oh, yeah.
HILTS: If I can add, too. I fully agree with both of you that sort of some of the fundamental privacy concepts that we’ve been dealing with traditionally need to evolve. And I think I agree again that, you know, the notion of, like, privacy rights at the time of collection versus the use, I think that is a more powerful way of looking at things.
And I think companies—and forgive me, that’s where sort of my research lies, is companies’ use of data, like consumer products. I think companies need to sort of adopt that as well in their own processes and policies. In my—so I’ve run a research project in Canada where we exercised our rights of access to personal data. So what we do is we write legal requests to companies asking them questions like, how’s my data being used? Have you disclosed it to third parties? If so, who? Have you disclosed it to law enforcement? And please provide me a copy of all the data that you have about me.
And in some of our findings, we definitely see that the data you get back is, you know, very much what personal data is stored in their databases, is retained in their databases. However, as Dan was mentioning, we see more and more of, you know, data that may not be stored, but it’s being processed and being used in real time to make decisions. And I think as a—you know, a citizen wanting rights of access, that sort of thing is a lot harder to get access to and to understand. And indeed, on the flipside is, I think—I would think harder for companies to provide access to that as well.
And I could give you an example. We sent access requests to online dating applications. And you know, we—one of the questions we asked is what sort of, you know, profiles have you grouped me into, like demographic or habitual or what have you. And we did not get any information back about that. What we did get back was sort of just a database dump, essentially. But we know, you know, from the use of these websites that you get filtered into certain groups and categorized and things like that. And, you know, presented to certain people and not to other people. And it is hard to be an informed consumer and understand how that’s actually happening when you can’t really get access to that information. And I think that’s quite a challenge and an opportunity as well.
BRUCE: Yeah.
CHENOK: I won’t comment on the dating issue. (Laughter.)
KORNBLUH: So I think it’s a good time to open it up to questions. And, wait, I have to read something when I say that. We’re going—it’s a reminder that this meeting is on the record. Please wait for the microphone and speak directly into it. Stand, state your name and affiliation, and please limit yourself to one question. Thank you.
Oh, come on, guys. Really?
Q: Good morning. I’m Michael Pocalyko. I’m managing director at Monticello Capital. And I also head our practice for fraud and investigations.
In three panels, no one has spoken about the relationship and tradeoffs of personal curation and fraud with respect to privacy. You mentioned in Canada we have seen a number of cases persons throughout executive life who are creating records that are fraudulent, are—or, to be more kind, a creation of a spun record. And the European right to be forgotten, as we heard before, are blocking out whole portions of persons lives that they don’t want to be. Try, for example, to find whether someone has actually been incarcerated in a public record, even in law enforcement databases today. You have to go county by county in that type of investigation. How is this imperative for privacy going to be impacting our ability to actually check whether someone is who they are?
HILTS: I do have a thought on that, if you don’t mind. So a project that I have been involved in looked at fitness tracking applications. And we do see, I think, a risk for fraud associated with some of the data that’s being produced by their devices and their companion smartphone applications. For example, there are several insurance companies that are exploring opportunities to integrate fitness tracking data into their offering so that, you know, you could get discounts on your premiums if you’re, you know, a daily Fitbit user and monitoring your step activity. And, you know, that on the surface seems pretty good.
However, there are, you know, risks associated with that. And fraud is one of them. In our research, we did technical tests on the data that’s being transmitted by these applications. And in several different instances with different companies, we were able to reverse engineer the APIs the these services used and were able to inject false records of taking steps. And we could have injected false records for many other activities too. So as a sort of—you know, a joke example, I was able to say that I took a billion steps in a single day. And, you know, that’s not realistic, but, you know, a more ambitious and potentially nefarious person could develop a tool to, you know, create some sort of seemingly natural-looking distribution of steps and submit that every day to this fitness tracking server to give the illusion that they were active when, in fact, they weren’t.
So this sort of reliance on data, particularly that’s generated by individuals using their phone, I think it does need to be taken with a grain of salt in terms of the integrity of the data. And Fitbit—to talk about solutions—they have one interesting way of combatting this tampering where—and in essence the wearable itself encrypted the records on the device and only Fitbit itself had the key to decrypt that data. So the mobile phone, while for other companies it was sort of the, you know, point of truth for the record keeping, it was merely a conduit for Fitbit so that the company had the sort of keys to the castle in terms of the integrity of the data. And I thought that was, you know, quite a clever way of getting around that problem.
CHENOK: There I was, thinking that I could tell my wife that I did a lot more exercise than I really did. (Laughter.) I think that it’s a really good point. Two observations. One, the same technologies can be used to detect anomalies in sort of algorithmic approaches and distributed technologies that can analyze data across multiple data sets, especially, let’s say, in the cyber area—can sort of detect anomalies in traffic patterns or can detect anomalies if you actually can capture multiple use instances across a financial portfolio and you can see sort of where somebody is making something that is coming from a different address, a different IP address, or a different authenticated user. And that can be spotted more quickly with some of these technologies. So there is the risk that somebody can create the false picture. There’s also the benefit to the technology of being able to catch that more easily.
The other thing I’d point out is that we think about applying this in combination with human decision making. I don’t know if you know about the example—the Canadian tax revenue example in Toronto, where they used data and crowd sourcing to understand who was trying to defraud the Canadian revenue authority from their nonprofit status. Are you—
HILTS: No, I haven’t heard it.
CHENOK: So basically they took a data picture of companies that were claiming tax exempt status, and they actually crowdsourced that because they were having trouble identifying where the fraudulent actors were, because they all kind of looked similar. So rather than try to sort of go transaction by transaction to determine whether an organization was fraudulently claiming nonprofit status, and thus improperly paying—or getting tax benefit as a result, they actually went to the community—the nonprofit community in Toronto and they said: Here’s the list of the companies that—or the organizations that are claiming nonprofit status. Based on our data that are coming in, can you tell us if you know these people?
And the charitable community came back and said, yeah, we know—I don’t know what the numbers were exactly—but we know this larger group, but this small group, we’ve not heard of these people. And it helped the authorities actually to target those organizations to determine whether or not they were charitable and thus tax exempt. It turned out that a number of them were not, and the Canadian authority was able to go back and go in and recover that fraud. So it’s an example of you take an algorithm and then you sort of use the wisdom of the crowd and open the data to result in a decision that could address your—an example like the one your raise.
KORNBLUH: Mark.
Q: Mark MacCarthy with Georgetown and SIIA.
I want to go back to the algorithmic fairness issue. And a first, just a comment on the Facebook situation. There was a difficulty that was pointed out in a ProPublica a couple of weeks ago. But it’s important to note that Facebook has already responded. They’ve put in place a decision to develop a tool that will identify when an ad is in the area of housing or employment or credit and then stop the use of the ethnic affinities categories in connection with those ads. And they did this in conjunction with discussions with interested groups, the Black Caucus, and civil rights groups, and so on.
And that leads me to my question, which is SIIA has put out an issue report on algorithmic fairness which suggests something I think that all of you have recognized, which is that fairness doesn’t happen by accident, it doesn’t happen automatically. It’s the kind of thing that has to be designed in. And, indeed, there needs to be a process of continuing monitoring to make sure that algorithms that are designed fairly are actually continuing to be fair and in actual use.
The question is, in designing this kind of process for fairness, is there a useful role for stakeholders from all sorts of different parts of the ecosystem, from industry, to consumer groups, to government officials, to sort of talk about these issues and design a sort of common understanding of what a system of fairness by design might look like?
BRUCE: Yes. (Laughter.) And I think—so, yes is the short answer. I do think to enable sort of these promises and optimism of big data and this kind of—I mean, the big opportunity here is to improve our decision making—we have to figure out privacy at scale. And I think it’s going to take a collaborative effort with—I think tech companies, to your point, with Facebook taking those steps, it’s like tech companies want to ensure there’s trust and they’re following these privacy principles, is my sense.
I sat at the table with a number of large companies, like Facebook, and they see that they’re sitting on a treasure trove of social science data. And I see people at MIT saying, oh my God, just give us the data. (Laughs.) We’d love to do some research on it. And that tension between Facebook saying we can’t just hand over all our data, right? We’re protecting—we’ve got privacy issues. They want to do—I feel like there’s a sense of wanting to do right by their users and that’s really important.
And on the other hand, I feel like the need to figure out the privacy solutions and the complexities that we’re all talking about around how do you know when you have algorithmic bias isn’t just, well, make your code available, because then we’ll know. (Laughs.) It’s a complex set of issues and I do think it’s going to take time and a dialogue between the tech companies, that have a lot of the technology solutions, and the policymakers and the civil society to say, well, what are our principles and what do we as a society—when you start asking these ethical questions—are really important to be having now because we’re deigning the systems now.
And I think that a lot of—you know, from being at MIT, there’s a lot of promise in technology delivering the solution to many of these things we’ve enabled. You know, you talk about encryption, talk about differential privacy, talk about, you know, anonymizing data. There’s a lot of kind of interesting technology solutions. Having sat through a lot of technical talks about all of them, there is no one solution. It’s not just encrypt all the data and we’re done.
That’s not it. And I feel like a lot of the conversation about big data has been about black and white, like, open data and private data. And we need to advance the conversation to talk about all the gray in between. And it’s layers of privacy, right? There’s all these shades of gray in between. It’s not just about making it public and open. There’s a lot of valuable data and ways that you can make data available that aren’t just about black and white, open and closed. And so that’s where I feel like we need to advance the conversation. And it’s going to require a multi-stakeholder discussion.
And so things like encryption, I think one of the exciting opportunities is that you can compute over encrypted data. So this is something in the labs. You know, it’s something called functional encryption. It allows you to keep your data encrypted, compute over it, and then calculate certain specific computations over that data that can be incredibly valuable. And that’s the same with differential privacy. What it provides is a guarantee—a mathematical guarantee of privacy.
These are things that when you have large—it’s not for I want to detect a terrorist or I want to target an ad at you, but it’s for those larger things that are, like, how could I improve the financial stability of, you know, our systems by computing aggregate systemic risk metrics over all of this financial data that, no, banks don’t want to give up all their data, but you could compute these specific metrics and it could be incredibly valuable to our economy.
So—(laughs)—that’s a long answer. But I’m saying there’s so much opportunity there, right, in that gray space. And those are the conversations I think we need to be having.
KORNBLUH: So I just want to pick up a little bit more on what Dan was suggesting with the discrimination issue. And, Dan, I don’t know if you have any thoughts about—I think what you’re talking about is the Facebook example and similar examples like that. And I’ve read Mark’s paper, which puts forward an idea of maybe doing disparate impact analysis at the end of the day. You know, have you thought about some of these fairness issues that you were raising before, and how business and government can work together to address some of them, and maybe some kind—or different private sector, maybe civil society and business. Maybe it’s not government.
CHENOK: Well, certainly, I also get—the answer is yes. There is opportunities for all sectors to work together in identifying solutions. I would say that, you know, there’s different pieces of privacy that come into play at different points of a transaction, or a piece of analysis by a company or a government agency about people that they’re serving. And, you know, fairness in process, the ability to kind of, again, take that principle and apply it to a world where data’s traveling in real time and giving notice to people where what—you don’t—in the old form of notice, it just kind of went out by mail, right?
And now it can go out in real time over a machine-to-machine interface, so that if a person sets up a privacy profile they have the ability to interpret fairness in their—based on their personal understanding of what privacy means to them. So they can basically be told every time something is happening about them in a particular company’s use of their information. They can sort of set up an alert.
So there’s different—the way I think about that is fairness as a general principle implied transparency and choice as well. And individuals in sort of the world of big data and open data and data that’s traveling across in real time, the ability of technology—and companies are looking to start to apply these kind of privacy notices, you talked about differential privacy—that can really create privacy profiles that can naturally use their needs, can be an important element of application of fairness at an individual level.
HILTS: If I can add to that briefly, I fully agree that there needs to be, you know, more transparency and, you know, giving individuals more access and control over what they’re consenting to. And you know, the sort of just, like, a way to manage what data is going out there about themselves. But I also have a slightly different view that with so much data out there, it is hard to grasp everything that’s happening about your data. And I think—but, I mean, at the same time, it is important to be transparent.
But I think we need, you know, alternate measures as well to ensure that whatever data is being used we are treating it accountably and fair. And that could be through—you know, not to make an argument for my own existence—(laughter)—but through, like, independent researchers who are verifying these claims and, you know—you know, impartial academic investigations, something like that. I think those also play a role in ensuring that this data is being used accountably and fairly.
CHENOK: Fully agree with the need for research. And I think that that kind of fits both companies, and users, and government.
KORNBLUH: And think tanks.
CHENOK: And think tanks.
KORNBLUH: Yeah. (Laughter.) Adam.
SEGAL: I wonder if you could speculate, because it seems to me that some of the biggest big data is going to be in Asia. And has there been any engagement or idea about how you get Baidu or Alibaba, or Tencent involved in these discussions, especially since the Chinese government seems to be interested in this plan to link all of social media data, purchasing data, with a kind of ideological measure about, you know, do you support the party? Have you spoken up? Can we funnel grants or loans, people that are—score higher on that? You know, what role do you think they’re going to play in shaping these debates? And is there a way that we can engage them in that?
HILTS: I can speak a little bit about Baidu, and that’s because at The Citizen Lab we’ve recently done an analysis of several Chinese mobile web browsers. And I can’t speak to, you know, Baidu as a whole company, but the browser in particular had a raft of severe security vulnerabilities in place, such as your geolocation being sent out with either no encryption or very weak encryption—like, nonstandard. It was like roll-your-own encryption. Keywords when you’re searching being sent with little protection. And a backdoor in the update mechanism where a man in the middle attacker could, you know, put in an arbitrary executable and take over your phone that way. So I would just definitely caution that, you know, while there’s a huge opportunity in China for big data, obviously, that some of the basic security practices need to, I would argue, be improved.
CHENOK: The other point I—rather than specifically focusing on one particular region—as a—we’re from both multinational companies that are dealing a lot with cross-water data exchange, which I know a lot of people in this room have thought a lot about as well. And so if you think—in that environment, we’re constantly looking at how do we design compute algorithms that are consistent with the laws and protections and cultures and norms of the particular region. And when the data is flowing across borders into servers, the question of how do we actually apply that to the software that we’re working on, to the systems that we’re developing, and into the services that we’re providing online is something that we have to constantly think about.
So as we look to developments in Asia and developments in Europe, and here in the U.S., we just need to be cognizant of both what are the legal frameworks, how do we harmonize those legal frameworks, and then what the culture expectations, which are more advanced in certain parts of the world where they’ve had a longer traditional of privacy protection, as in Europe.
KORNBLUH: And where international norms, as an American company, you will abide by. Yeah.
Q: Thanks very much. I’m Heidi Tworek. I’m a fellow at the German Marshall Fund this year.
I wanted to ask a much broader question about fairness, which is the question of what actually has the ability to create data in the first place. So the World Bank data tells us that internet penetration rates in the U.S. as of 2015 was 74.5 percent. So the question is, when you have machine learning that is based on only 74.5 percent of your population, how do you account for the other 25.5 percent? And what are the problems here of machines learning from only three-quarters of your population, leaving out particularly people in rural places? Is there any way that these companies or academics are thinking about combatting this absolute fundamental bias in the way that machine learning occurs?
BRUCE: Well, I can’t speak to specifics, but I know in general companies are trying to get the internet and computing everywhere in the world. So there’s progress on trying to make sure that everyone is, you know, part of the global internet society and can access. And phones—mobile phones are everywhere. So I think that will just continue to progress. I’ll say that I feel like the—you know, there’s definitely bias. And that bias comes from the data. So if you look at, like, Twitter, and you’re making inferences off Twitter data, you’re looking at a certain population. And only a certain amount of Twitter data is geocoded. So again, you’ve got bias in the subset of sample of data that you’re looking at.
There’s always going to be bias in the data, I think. I’m not sure you’re going to collect data in the future that’s completely unbiased. So I think the people designing the algorithms need to be intelligent about thinking about the biases that are inherent in the data that those algorithms are learning from. I don’t know if that answers your question but—yeah. I think there will always be bias in the data.
CHENOK: Well, and I think it’s a real concern, about as we are gathering information are there segments of populations that are just not being reflected.
BRUCE: There will be.
CHENOK: Thus, decision making is going to be suboptimal, because you won’t have information about those populations that are in need. And especially when thinking about sort of government-serving populations who have various different levels of disadvantage and government programs are designed for them, they may not naturally have the same kind of access. So it is imperative on governments, on companies working with governments to think about that and to I guess sort of—I don’t—maybe oversample may be the wrong word, but to sort of be cognizant of reaching out to apply information about those populations and also, over time, shrink the impact of that population in terms of closing digital divides around the world.
HILTS: I would just add about crowdsourcing, and that that has the same issues. You might think that, oh, I’ll let the people collect the data and it will be, you know, representative. But in fact it depends on a lot of factors, not just limited to, you know, just having access to the internet. It also comes with, like, digital literacy and sort of your socioeconomic status. Do you have time to even participate in this? So there’s so many things to just be mindful of. It’s, you know, a challenge and I think, you know, a valuable one to undertake.
KORNBLUH: Yeah. I think we have time for one more question. Yeah, here. We can do two.
Q: Thank you.
So going back to the problem of detecting adverse impacts of algorithms on vulnerable populations, we talked about the increasingly less-useful nature of, like, requesting what data a company has, like, on your profile. What about looking at the algorithmic problem from a—from the other side of the black box, and asking them for what they—how they sorted you? So it’s less important what their algorithm looks like, because I understand that’s their secret sauce. But it’s much more interesting to understand where you sort of fall out in their data. I’d be interested in, like, whether you think the business community would be open to that, either from requests from individuals and also from requests for think tanks, or both.
HILTS: I can speak to that quick, in that in our research we did ask that question about online dating applications. And companies did not, you know, provide that sort of detail. And I mean, that may be because it’s sort of a real-time calculation and they’re not—you know, that’s not part of their data access process to do those calculations to provide access. They’re just, I don’t know, doing, like, a select query on the database. So I think—I think company processes need to become more mature to the changing definitions of privacy and how—and what, you know, an informed consumer wants to understand.
CHENOK: I think that goes back to the use scenario that we talked about earlier around, yeah, companies are going to do a lot with information, but it’s at the point of making a decision about a service that a customer is asking for, or a government agency making a decision about a benefit or an enforcement action. And at that point, there needs to be, obviously, clear understanding by the individual about what’s happening, access, even if the data is traveling so quickly that it’s hard to sort of implement that in real time within, as you call it, the black box, as they’re going through that. So it comes back to the point about—that the President’s Commission on Science and Technology talked about a couple years ago, that the Australia report talked about, about moving to this sort of use paradigm as opposed to a collection paradigm.
KORNBLUH: I think we have one more question here.
Q: Thank you. Jim Hoagland, Washington Post.
Andrew mentioned the need to continually monitor algorithms. And I wonder if you could talk about who should do that monitoring? And if the other panelists would also chip in on the question of how you see the evolving regulatory and legislative framework for the use of big data.
HILTS: Yeah. I can speak to—at least, I think that there is a need for independent research to be done on companies. I mean, I would hope that companies have the best intentions of, you know, ensuring fairness in their algorithms. But I think especially when it’s making decisions about people’s livelihoods or their opportunities, that there does need to be an independent organization, whether that’s an academic research group or sort of a nonpartisan NGO that does this sort of work. But I mean, if it—if sort of the algorithms are in this, you know, black box, it’s hard to really understand what’s happening. So the most you can do is sort of, I guess, a post hoc analysis, like ProPublica did, in sort of getting access to some of the scores that convicts had comparing that to their rates of committing crime again. Yeah.
BRUCE: (Laughs.) My only response to that is that we need to come up with something scalable if we feel like monitoring algorithms is an important function to ensure fairness. You know, as wonderful as all the, you know, academics who produce these kinds of—and independent. You know, and they do the best research and uncover potential bias. That’s one way of looking back and saying: This isn’t right. But we need to have something—I think this kind of idea of privacy at scale needs to have something that’s going to keep up with the rate at which algorithms are going to be making decisions in our lives.
CHENOK: I would say this concept of privacy by design, which was talked about 20 years ago, also applies to sort of a new world of cognitive and algorithmic computing. And understanding how to build in—where PII can be implicated at a particular moment in that chain, and how to address that responsibility in the systems that are being developed is something that companies are certainly working toward. And from a law and policy perspective, as in many places, law and policy may not be sort of where the technology interfaces with the user community.
And again, you’ve got a privacy act in the U.S. that was written in 1974. The principles are enduring. But I think about—it was about seven years ago the Center for Democracy Technology worked with Senator Akaka to promote a revision of the Privacy Act to sort of take into account new technologies. And I think that efforts like that to kind of take a look at law and policy and the framework that applies to the new technologies is a useful exercise.
BRUCE: Can I just add, just to try to be, end on a positive note, is I do think a number of these emerging technologies—so, we have things like differential privacy and new technologies that’ll enable you to derive some of the benefits of big data without revealing individual personal privacy. I also—there’s an interesting emerging area right around policy as code. If you think of law as a series of—you know, it is code in some sense. You’re writing a policy into a set of practices. There’s working going on where you could, say, computationally prove that some data has been stored and accessed and used under HIPAA compliance. You’ll—(end of available audio).
(END)
This is an uncorrected transcript.